*****************************************
Abstract
We train an object detection model on a large dataset with 4,700 diverse high-resolution RGB images containing 190,000 labeled wheat heads. The trained model is the YOLOv5x object detection model, which utilizes one-stage detectors for dense prediction and a secondary stage to select certain regions of interest (ROIs) to maximize efficiency and accuracy. During training, we opt to utilize the Global Intersection over Union (GIoU) loss function to maximize our model’s holistic training. By doing so, high mAP@.5 and mAP@[.5:.95] values of 93.8% and 53.83%, respectively, are achieved. These metrics are comparable to many state-of-the-art models used on datasets such as the Common Objects in Context (COCO) dataset, showing our model to be quite effective. Additionally, our great model robustness is also communicated through our model’s high accuracy and low loss values. This work is significant in improving agricultural technology, allowing for the future automated surveying of crops to reduce cancers from sunlight and pesticide exposure.
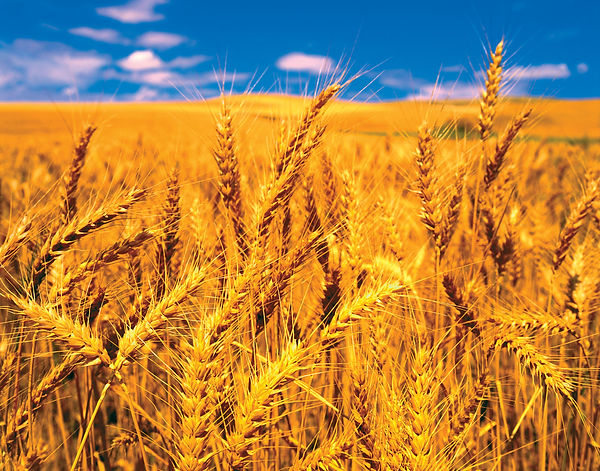